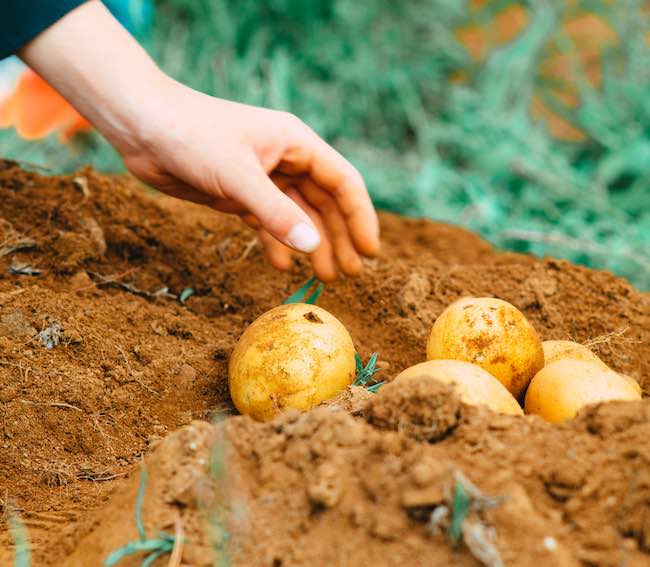
Agriculture plays a crucial role in the UK’s economy and provides an essential source of food for the population. However, crop production is affected by various factors, such as weather, light conditions, pests, diseases, and soil conditions, which can lead to crop failure and reduce yields. An AI and data-driven approach can help to mitigate these risks and increase crop yields by enabling more precise watering, nutrient application, and early detection and prevention of pest and disease attacks. This project aims to develop an AI and data-driven system to enhance crop security in the UK.
Methodology: The proposed system will leverage multiple sources of data, including climate, weather, air and soil temperatures, crop growth stages, soil moisture content, nutrient levels, and pest and disease occurrences. Machine learning algorithms will be used to analyze these data sets and identify patterns and correlations that can inform the decision-making process.
The system will be designed to provide farmers with recommendations on when to irrigate, fertilize, and apply pesticides based on real-time data, enabling more precise and efficient use of resources. Additionally, the system will be able to forecast pest and disease outbreaks, allowing farmers to take preventive measures before they occur.
To develop this system, a team of AI and data science experts, agronomists, and farmers will collaborate to collect and analyze data, develop and test machine learning models, and deploy the system in the field. The project will be carried out in several phases:
Phase 1: Data collection and analysis In this phase, the team will collect historical and real-time data on climate, weather, air and soil temperatures, crop growth stages, soil moisture content, nutrient levels, and pest and disease occurrences. The team will then perform data cleaning and preprocessing to prepare the data for analysis.
Phase 2: Model development and testing In this phase, the team will develop machine learning models to analyze the data and make recommendations on watering, nutrient application, and pest and disease prevention. The models will be trained and tested using historical data and validated using real-time data from field trials.
Phase 3: Field trials In this phase, the system will be deployed in the field, and farmers will use it to make irrigation, fertilization, and pest and disease prevention decisions. The team will collect data on the system’s performance and make any necessary adjustments to improve its accuracy and effectiveness.
Phase 4: Deployment and scaling In this phase, the system will be refined based on the results of the field trials and deployed to a larger number of farms. The team will provide training and support to farmers to ensure they can use the system effectively and optimize their crop yields.
Expected outcomes: The proposed system will enable farmers to make more precise and data-driven decisions on when to water, fertilize, and apply pesticides, leading to more efficient use of resources and higher crop yields. Additionally, the system’s ability to forecast pest and disease outbreaks will enable farmers to take preventive measures and reduce the need for reactive treatments, leading to a reduction in the use of pesticides and improved environmental sustainability. Finally, the project will contribute to the development of AI and data-driven solutions for agriculture and provide insights into how such technologies can be deployed to enhance crop security and increase food production.